Is ego depletion real?
My notes on Is Ego Depletion Real? An Analysis of Arguments. Malte Friese, et al.
This paper looks at the ego depletion research to see if there’s enough evidence against ego depletion to convince a proponent that it does not exist, or if there’s enough evidence for ego depletion to convince a skeptic that it does in fact exist. The conclusion of the paper is that the available evidence is inconclusive, and can’t ultimately make a compelling case on either side of the debate.
(These are my personal notes, so they may not all make sense to you.)
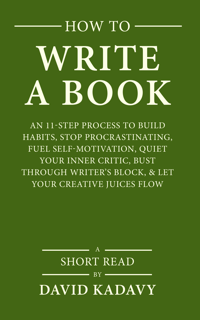
WANT TO WRITE A BOOK?
Download your FREE copy of How to Write a Book »
(for a limited time)
What is ego depletion?
- Baumeister et al. concluded that self-control is independent of domain. So if someone has poor self-control in their dietary habits, they’ll also have poor self-control in their exercise or relationship habits.
- They also concluded that self-control saps your energy, which then makes it harder for you to exhibit self-control later on.
- So, the ego depletion hypothesis is that if you exert self-control on one thing, you’ll later struggle with self-control on another thing.
- But the idea has been broadened over the years to include things outside of self-control. So if you do a mentally demanding task or make a decision, you’ll later have trouble doing any of these other tasks falling under this wide umbrella.
- The idea of ego depletion comes from the strength model of self-control. The idea being that self-control comes from a resource that applies to many different domains.
Alternative models
- There are some models that explain this ego depletion effect through other constructs, such as motivation. (Inzlicht & Schmeiche, 2012; Kool & Botvinick, 2014; Kurzban, Duckworth et al., 2013)
Evidence against ego depletion
- Hagger et al. (2016) did a large-scale replication (RRR) of one study and found no effect.
- Hagger et al. (2010) did a meta analysis of ego depletion studies, and found an effect. But then Carter & McCullough (2014) reanalyzed that data and found publication bias in the data. Meaning that unpublished studies weren’t included in the data, so of course there was an effect.
- Yet another meta-analysis included unpublished studies, and found an effect. But then when they corrected for publication bias through various methods, the results were inconclusive. (Presumably there’s still a chance of publication bias when you include unpublished studies.)
- Aside from publication bias, there’s also a lot of p-hacking going on in psychology research. This includes only including the depending variables that “worked”, while leaving out the others, deciding to include or exclude data to find an effect, peeking at the data to stop when the desired effect is seen, or including covariates without a good reason.
In defense of ego depletion
- In the paper, they attempt to defend ego depletion in six ways:
- Explaining the limitations of meta-analysis and replication (RRR)
- explaining the shortcomings of the manipulations and dependent variables used in ego depletion research
- moderator and mediator studies
- there isn’t much evidence of “reverse-depletion”, or performing better after a prior task
- analyzing the size of the hypothetical “file drawer” (I guess studies which didn’t get published)
- presenting abundant evidence for ego depletion in everyday life
Limitations of meta-analyses
- Carter et al. (2015) did a meta analysis of ego depletion research. They took 620 studies, and reduced them down to 116 studies, by only choosing studies that shared the most common variables in their methodology.
- By making this restriction, they hoped to reduce the data only down to the most direct possible tests of ego depletion.
- But in doing so, they reduced any chance for boundary conditions.
- Another problem with the study was that one fourth of the studies were conducted by a collection of 10 graduate students, who didn’t have a lot of research experience. These were unpublished theses and dissertations, and naturally they had smaller sample sizes and lower statistical power.
- The procedures used to correct bias in the ego depletion literature are themselves biased.
- Trim-and-fill assumes that publication bias is because of weak effects, and not significance. It also assumes that effects all gather around one “true” fixed effect. Finally it assumes any asymmetry in the “funnel plot” is caused by publication bias, rather than other possibly mundane effects associated with small studies.
- Both PET and PEESE produce estimates that vary in conditions that aren’t unusual for psychological research (heterogeneity, p-hacking, and publication bias). So, this causes undercorrections and overcorrections.
- Selecting based upon p-value assumes effect sizes are the same across studies, that statistically non-significant studies don’t matter, and that significant studies are equally likely to be published if they have equal p-values.
Limitations of replication studies (RRR)
- Hagger et al.’s analysis showed no reliable effect between the digitalized e-crossing and the MSIT.
- But remember that ego depletion research is inherently wide in that there are a variety of manipulations and variables across the many studies. So, showing no effect in this one study does not disprove the entire theory.
Shortcomings of manipulations and dependent variables
- Hagger et al. analyzed 198 ego depletion studies (2010) and found that only thirty percent of them asked about the difficulty of the first task, fifteen percent asked about subjective effort, and twelve percent asked about fatigue.
- If the theory is based upon exerting self-control, you need to measure that somehow, but many studies aren’t doing that!
- Many of the manipulation conditions aren’t likely to cause much of a motivation shift. Compare these with things we do in everyday life that require a lot more effort.
- The outcome variables vary widely across studies. Some examine risk-taking behavior, achievement motivation, persistence in doing a puzzle, hand grip, unhealthy behaviors such as easy junk food, drinking alcohol, or smoking – amongst others!
- These variables were likely to have been chosen by what researchers thought would work. The data would be more robust if these variables had been randomly allocated to studies. This would support that idea that all of these things are drawing from a shared resource.
Limitations of Moderator and Mediator studies
- Moderator and mediator studies are simpler than simple “control vs. depletion” condition studies. This makes false positives less likely. So, moderator and mediator studies could be more robust.
- Incentives to perform well on the second task moderated ego depletion. This included financial or social benefits (i.e. that doing well will help others)
- Watching a funny video or getting a gift moderated any ego depletion effect. But being put into a neutral or sad mood did show an ego depletion effect.
- High construal level and having implementation intentions also counteracted ego depletion.
- People who believe that willpower is limited show ego depletion. People who don’t, do not
- Follow up (Job, Dweck, Walton 2010)
- Action-oriented vs. state-oriented people: action-oriented don’t show depletion effects because they keep allocating resources. State-oriented conserve strength (Gropel, Baumeister, & Beckman, 2014)
- Seeing emotion suppression in an EEG led to lowered performance on follow-up task. Exerting effort may impair monitoring process of error-related negativity
- Follow up: (Inzlicght & Gutsell, 2007)
- Mediator studies are harder to p-hack than even moderator studies. The condition has to correlate with both the dependent variable and the mediator.
- But, hardly any published depletion studies have been preregistered, which means that we don’t know how many mediator/moderator studies were assessed but never reported.
- It’s surprising there aren’t more studies that look at underlying mechanisms and test for statistical mediation. Especially with how much ego depletion research is out there!
Absence of Reverse Depletion Effects
- If there were indeed no ego depletion effect, then all of the data should scatter around “zero effect.” Thus, there should be hundreds of studies showing reverse depletion effects. In other words, showing increased performance after doing something requiring self-control.
- They show 1,000 fictitious studies showing no effect, but statistical variation, scattered on a funnel plot. They then account for various levels of p-hacking or publication bias.
- P-hacking would shift some studies with weak effects into the territory where they show an effect.
- Publication bias would exclude some of the studies not showing a significant effect, thus once again skewing the overall data toward showing an effect.
- The authors estimate (in one “reasonable scenario”) that there would be around 190 studies showing significant reverse depletion effect – in the form of false positives – if there were indeed zero effect.
- Yet surely journals would be eager to publish studies showing a reverse effect.
- So the question is: How big is the “file drawer” – the number of studies that didn’t get published – perhaps because they didn’t show a significant effect, or because they weren’t submitted.
- They go through a number of scenarios trying to determine how big the “file drawer” might be. If there are 750 ego depletion studies showing an effect. If there is in fact no effect, then about 75% of those would have to be p-hacked, and 25% would have to be false positives (it should be 2.5%). The file drawer would have to be filled with about 7,000 studies.
- Based upon the number they put in their simulation 0.2 = in danger of p-hacking. Chance of p-hacking 50%
- If they assume there actually is an effect, the more pronounced that effect, the smaller the hypothetical “file drawer” gets. So if they assume an effect of 0.3, the file drawer shrinks from about 7,000 to 1,300 studies.
- They go through a number of scenarios trying to determine how big the “file drawer” might be. If there are 750 ego depletion studies showing an effect. If there is in fact no effect, then about 75% of those would have to be p-hacked, and 25% would have to be false positives (it should be 2.5%). The file drawer would have to be filled with about 7,000 studies.
Evidence for Ego Depletion in everyday life
- Caregivers working in hospitals washed their hands 9% less by the end of their typical work shift. There was a more pronounced effect when work intensity was higher, and taking breaks reduced this effect. (Dai et al., 2015)
- Doctors prescribed antibiotics at a higher rater leading up to lunch. The same pattern repeated after lunch into the afternoon. (Linder et al., 2014)
- Danish students did worse on their tests the later in the day they were tested. But a 20- to 30-minute break improved performance. (Seivertsen et al., 2016)
- Consumers who made complicated decisions later chose default options more. (Levav et al., 2010)
- Putting an issue later in the ballot made it more likely people would abstain or choose the default option. It also made it more likely they’d vote for the first candidate. (Augenblick and Nicholson, 2016)
- College students who needed more self-control daily got into more arguments, studies less. (Simons et al., 2016)
- Students with more self-control demands were more likely to violate their personal drinking limit (Muraven et al., 2005)
- Employees with self-control demands were motivated to regulate their affect, and in turn ate more sweet snacks (Sonnertag et al., 2017)
- The more likely someone tried to resist a desire, the more likely they later succumbed to that desire (Hofmann et al., 2012)
What does this all mean?
- It would help if scientists preregistered their studies. This would prevent some p-hacking and reduce the size of the “file drawer.” (a framework called “Open Science”)
- Sample sizes should be larger. That would increase statistical power.
- “Sequential stopping” procedures should be employed. This would allow researchers to “peek” at results mid-study, but would also account for the implications of that peeking. Researchers could then decide whether it was worth continuing the study, which would save resources.
- Follow up: Bayesian statistics (Schönbrodt, Wagenmakers, Zehetleitner, & Perugini, 2017)
- A big source of the replicability problems is lack of sound theory. It’s like they’ve collected a bunch of bricks (individual studies), but they don’t have a plan for the structure of the house (theory).
- Researchers should focus on the amount of control required by a manipulation. Control number of dominant responses required, assess exerted effort, or go off self-reports about the subjective control the task requires.
- (Still feels subjective. Like wouldn’t a person more likely to report something took self-control also be more likely to experience ego depletion?)
- Researching mediating effects is helpful, but it also conflates the functional with the cognitive. It’s possible that a mediating process is caused by the exertion of control, but it’s also possible something else caused it. So, the authors propose that manipulation checks focus on what’s causing the effect, and be independent of the proposed mechanism.
- “Strength model” would need to show evidence of diminished resources.
- “opportunity cost model” would have to show cost-benefit computations.
- Kurzban et al., 2013 (looks like they argue that making cost-benefit calculations leads to the draining effect of a perceived pointless task)
- “process model” would have to show reduced motivation, which would be very hard to assess.
- All of these are hard to measure!
- The theory doesn’t make it clear how these qualities interact with the “causal antecedent” such as exerted self-control.
- Theories talk about effort, but what kind of effort?
- Maybe they could objectively asses this through blood pressure or pupil dilation.
- Or maybe it’s all about subjective effort, and it wouldn’t show up psychophysiologically.
- One example of “building a building” or “inductive” theory building was the development of the Big Five.
Comments on methods
- Meta analysis have a problem in that p-hacking and publication bias skew their results.
- Publication bias is probably a bigger problem than p-hacking. Publishing even a small portion of insignificant studies would have a big impact on how skewed meta analysis results are likely to be.
- If 50% of studies in danger of being p-hacked are p-hacked, but no insignificant studies are published, that boosts the effect by 0.61.
- By simply publishing 5% of the insignificant studies, that boost is only 0.39.
- By publishing 30% of the insignificant studies, the boost becomes just 0.12.